Our cases
- Industry
- Areas of AI
- Capabilities
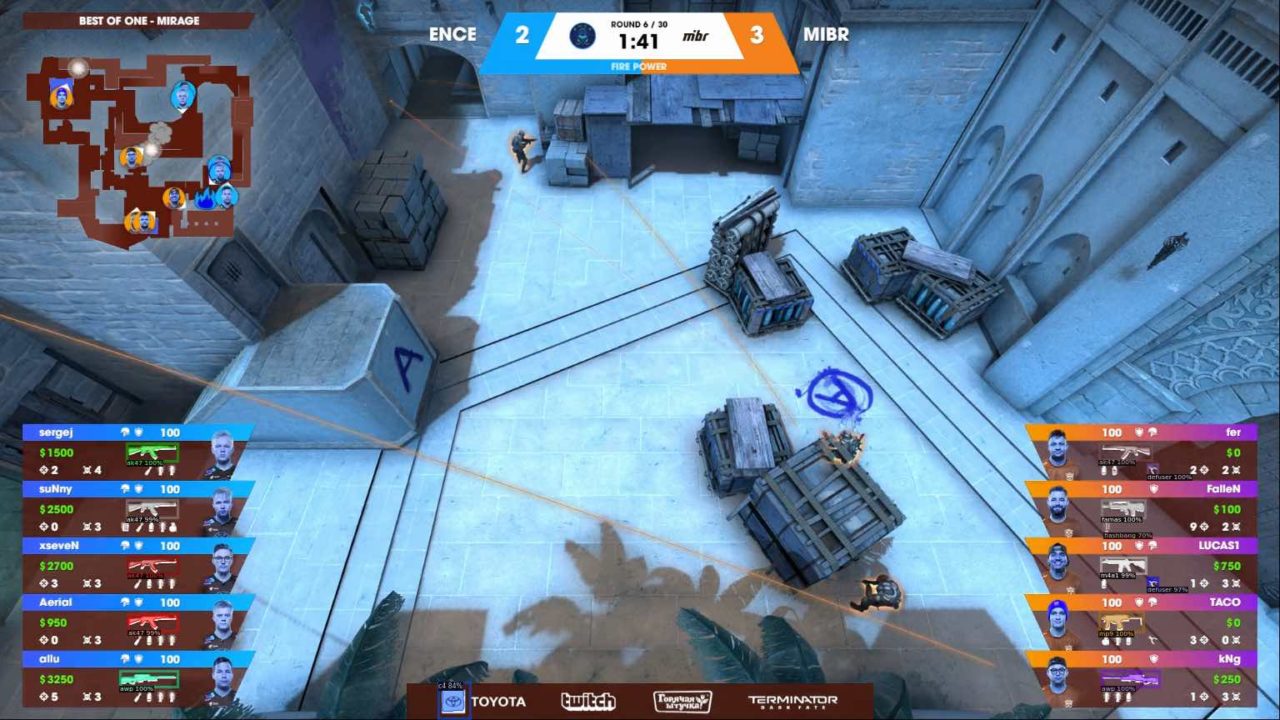
Real-time object detection for online gaming tournaments
Abios Gaming is a startup that focuses on tracking real-time events in online gaming tournament video streams. They asked us to improve detection performance and make previously impossible detections, possible.
Among the objects to be tracked were various weapons and tools the players use in-game, as well as certain interactions. The system detects and tracks timestamped events in correspondence with the objects the players possess, or use, to provide a structured stream of events that captures essential information about the evolution of the tournament.
Read morePredicting when rented equipment will be returned
As one of the largest rental equipment providers in the Nordics and suppliers to thousands of construction sites, this client needed a system capable of predicting when lent out equipment was expected to be returned.
The goal was to optimize the vast logistics chain by minimizing unnecessary transports between their local shops and main warehouses.
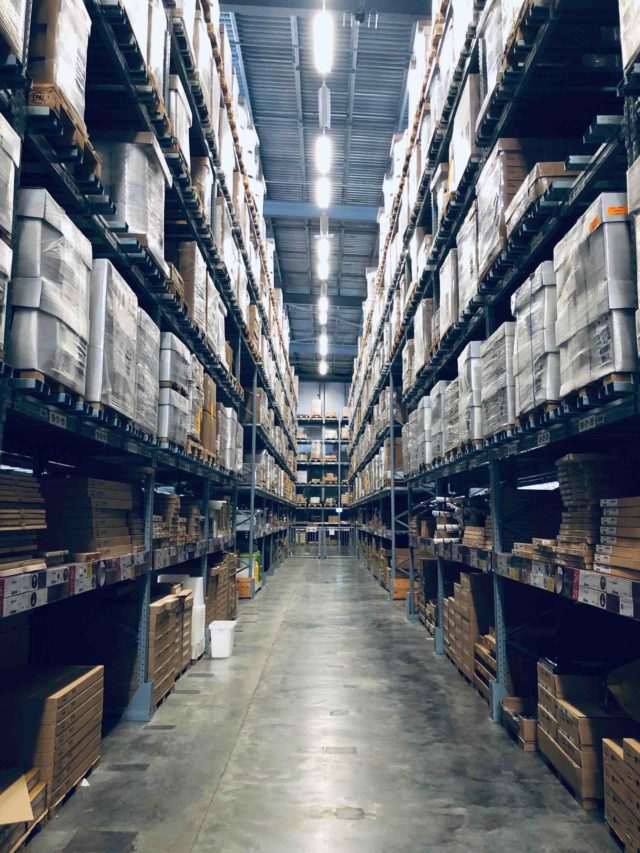
Detecting underlying paroxysmal atrial fibrillation for Zenicor
Together with Zenicor Medical Systems AB, we trained a deep learning model to predict an underlying paroxysmal atrial fibrillation (AF) condition in patients.
Every day, thousands of people suffer from stroke due to an underlying undiagnosed AF condition. Stroke often results in conditions such as paralysis or, in many cases, death. For this reason, precise and early diagnosis of AF is an essential part of stroke prevention.
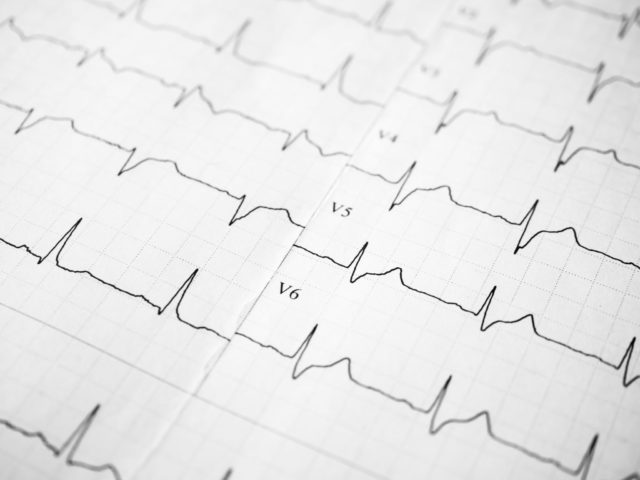
Detecting food intake in glucose time-series from diabetes patients
Together with Digital Diabetes Analytics, we created a meal detection system using glucose level data to help people with insulin-treated diabetes.
Today, more than 5,5 million people worldwide live with type 1 diabetes and need insulin-based treatment to survive. When it comes to diabetes, there’s no “one treatment fits all”, therefore doctor’s need support in optimizing treatments for each patient.
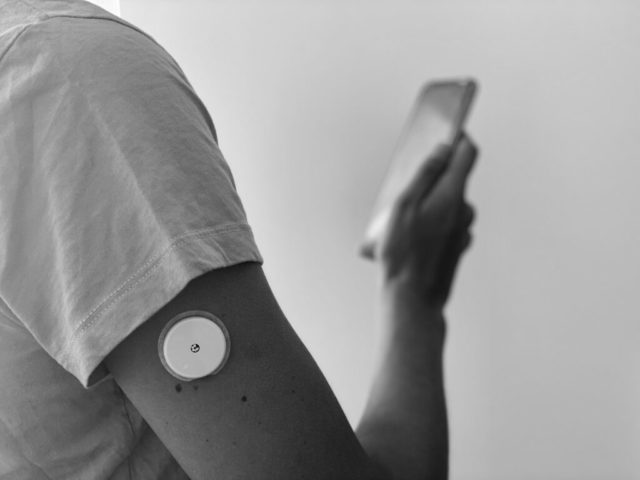
Personalized recommendations for Lindex
Lindex is dedicated to offer their customers a relevant and transparent personalized experience, in a multi-channel context. To be able to deliver on that front, a robust recommender system is considered a vital cornerstone.
Lindex is a major retailer, active in the Nordics and throughout Europe. They have several million registered customers, and is one of the prominent brands in women’s’ wear, lingerie and kids wear. Nowadays, users expect retailers to provide them with recommendations based on what they’ve clicked on and bought. A feature that both generates more sales and provides the e-shopper with a sense of being cared for.
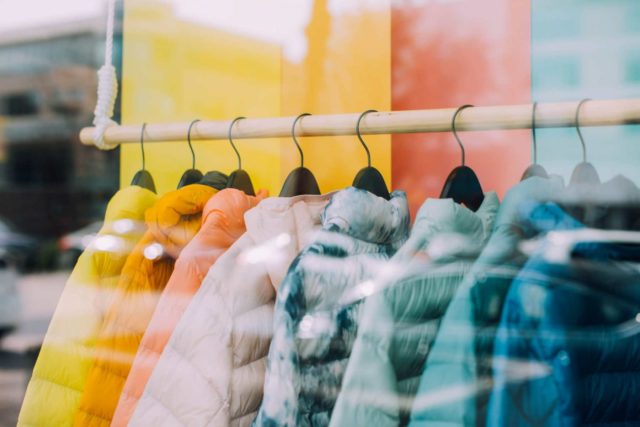
Forecasting the success of fishing trips, for millions of Fishbrain users
Data from 2.5 millions catches was collected and refined. Each data point was annotated with date, time, location, and fish species. Detailed weather information was added to each catch; air temperature, air pressure, wind speed, cloud cover, and precipitation. Temporal data was transformed into astronomical conditions such as moon phase, solar irradiation, and azimuth angle. A global climate model was created,
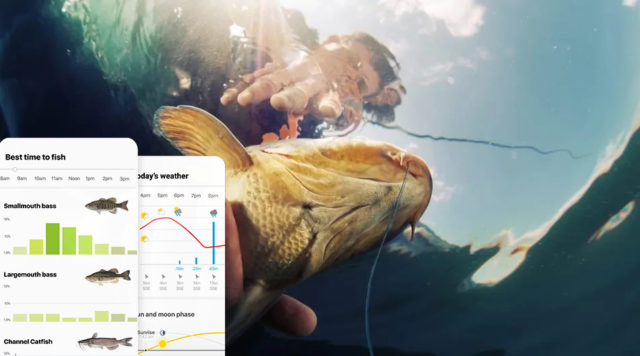
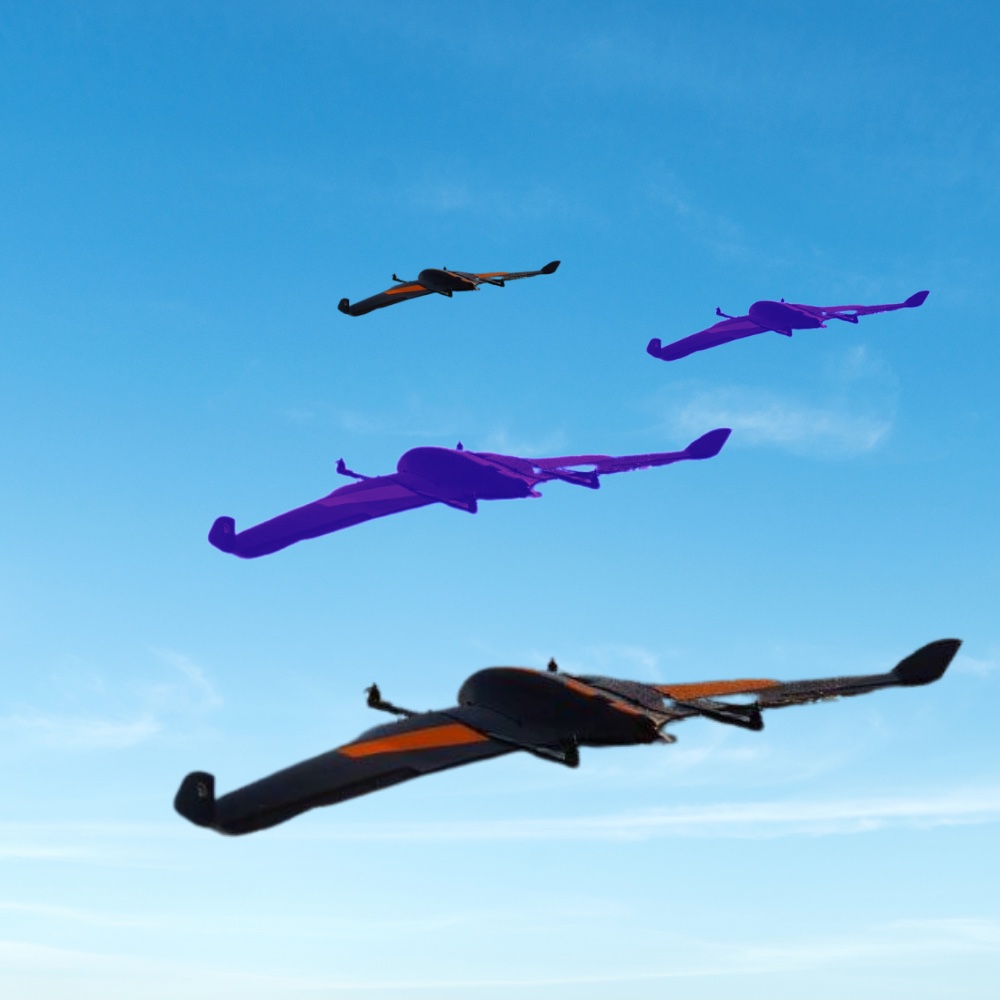
Detecting faulty transmission pole guy wires in drone images
The system consists of deep learning and traditional machine learning parts and is able of screening vast amounts of drone images to detect faulty guy wires.
The objective of the project was to create a machine learning pipeline to analyze images from power lines and detect lack of tension in guy wires. To solve this problem, the team broke down the problem in different stages and created a multi-model AI solution, in close collaboration with the client.
Read moreProduct recommendations for Ahlsell’s website and app
We helped out with kickstarting the in-house AI capabilities at Ahlsell by collaborating on the development of a recommender system for their website and smartphone app.
In close collaboration with Ahlsell’s data scientists, the team developed an end-to-end pipeline handling data ingestion, data processing, and model predictions. The recommendation system consists of a collaborative filtering model as well as a content-based model.
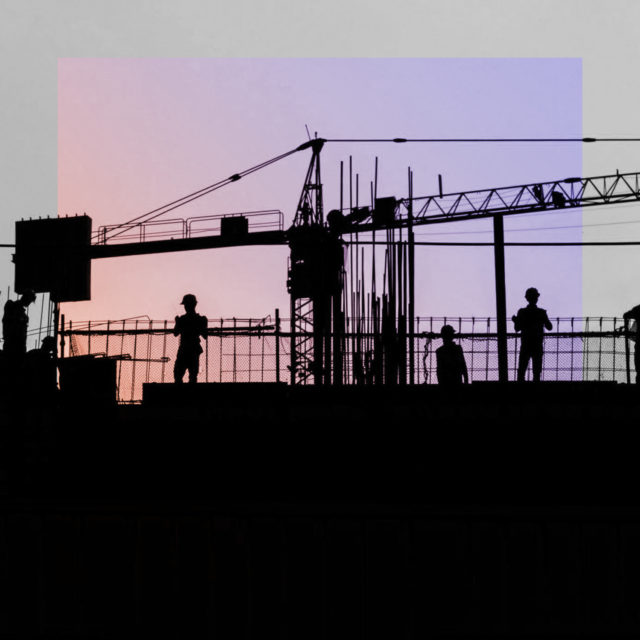