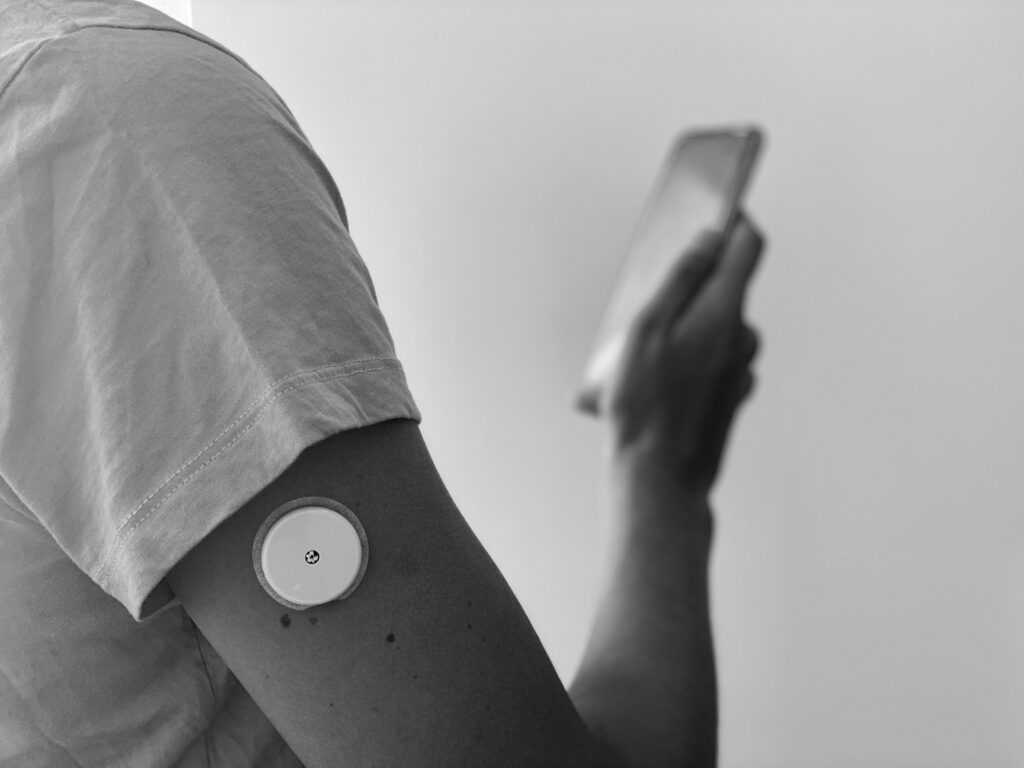
Detecting food intake in glucose time-series from diabetes patients
Detecting food intake in glucose time-series from diabetes patients
Together with Digital Diabetes Analytics, we created a meal detection system using glucose level data to help people with insulin-treated diabetes.
Background
Today, more than 5,5 million people worldwide live with type 1 diabetes and need insulin-based treatment to survive. When it comes to diabetes, there’s no “one treatment fits all”, therefore doctors need support in optimizing treatments for each patient.
Solution
Together with Digital Diabetes Analytics, we created a deep learning-based system that uses data generated by continuous glucose monitoring (CGM) to detect meal intakes. This system supports physicians in better analyzing large amounts of information about glucose levels and insulin administration, helping them optimize treatments. Meal identification may also help tackle other related issues such as Ghost carbs, a phenomenon where patients circumvent a badly tuned treatment plan by falsely reporting food intake. The solution helps physicians analyze large amounts of data from the CGM-sensors to recommend treatments and support people with diabetes. Furthermore, the meal-detection system helps reduce the error from manual meal intake-annotation.
Tools/Tech
We worked closely with the client’s health experts to verify and analyze data from CGM sensors and patient-annotated meals. Using time-series data, the team created a CNN-based model that predicts when a patient had a meal intake. The model training and testing were created using open-source libraries such as Tensorflow.
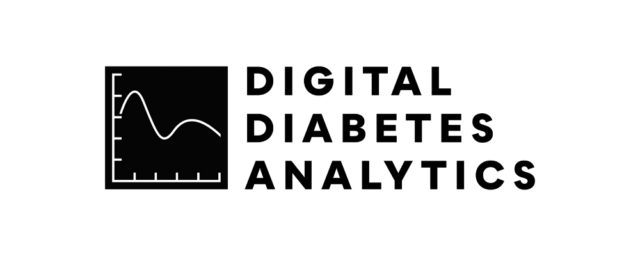
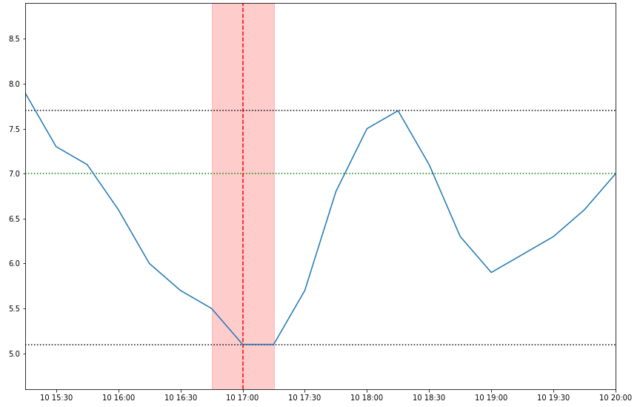
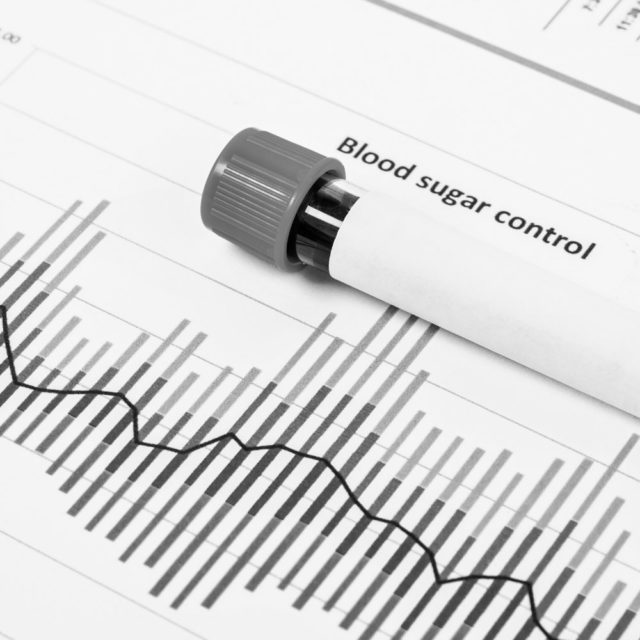
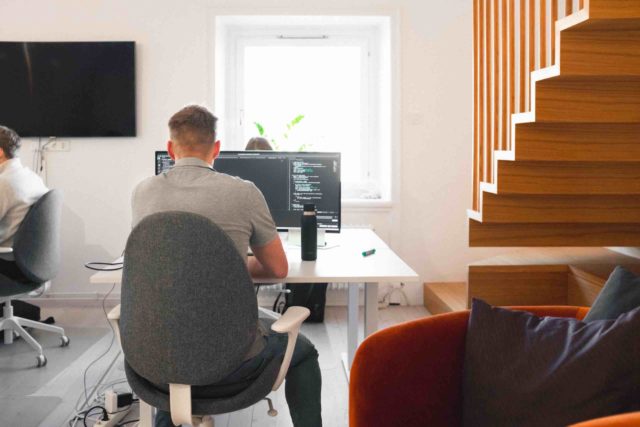