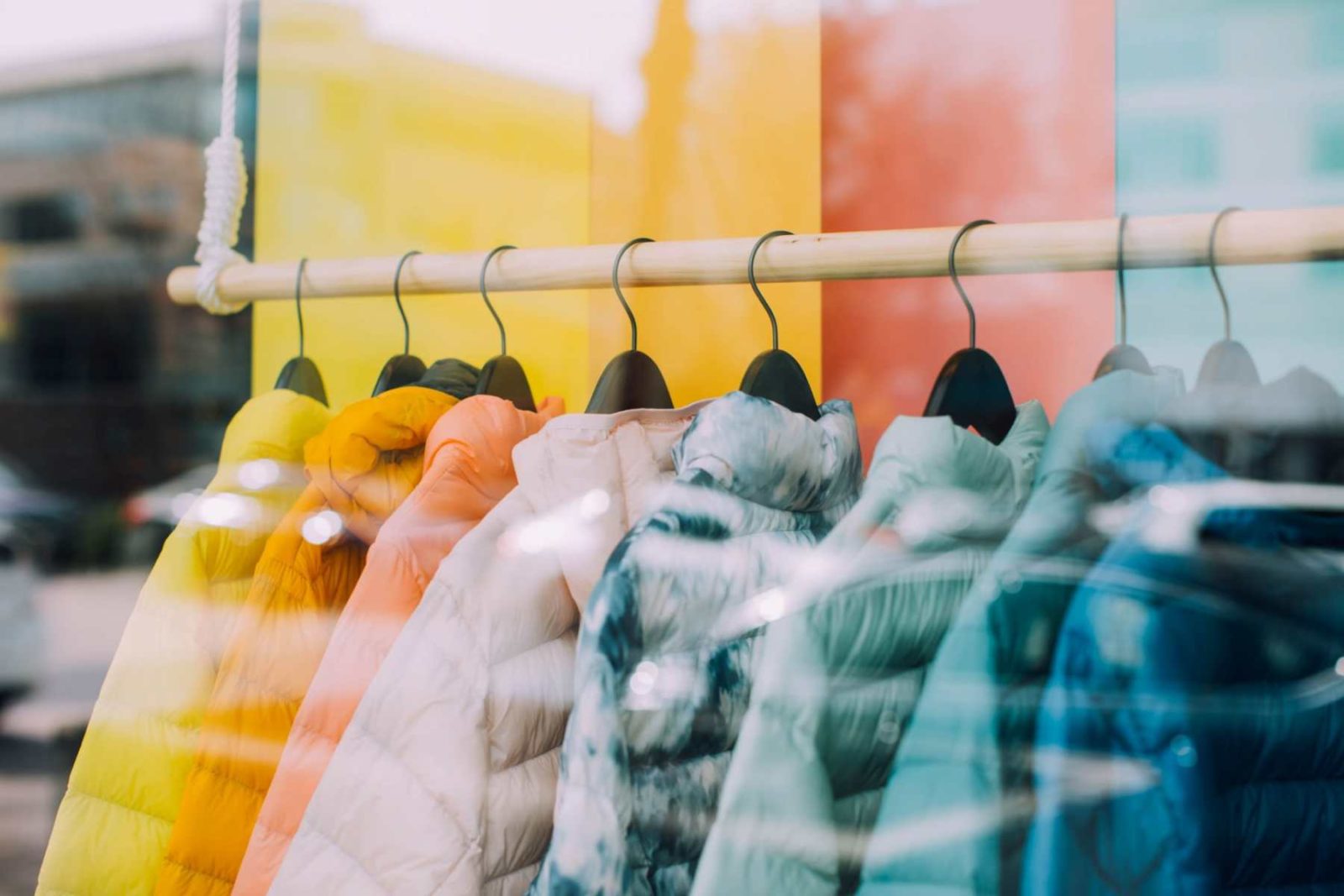
Personalized recommendations for Lindex
Personalized recommendations for Lindex, one of the largest retailers in the Nordics
Modulai has, in collaboration with Lindex, developed and deployed a custom-built recommender system. Lindex is dedicated to offer their customers a relevant and transparent personalized experience, in a multi-channel context. To be able to deliver on that front, a robust recommender system is considered a vital cornerstone.
Background
Lindex is a major retailer, active in the Nordics and throughout Europe. They have several million registered customers and are one of the prominent brands in women’s wear, lingerie, and kidswear. Nowadays, users expect retailers to provide them with recommendations based on what they’ve clicked on and bought. A feature that both generates more sales and provides the e-shopper with a sense of being cared for.
Solution
Data on approximately 60000 products and purchases from over 2 million customers was used. Customers were characterized using their historical transactions. Various sets of data were considered for feature extraction, including multiple levels of product categories, product attributes such as color, texture, and material. Natural language data was included and processed using various classical NLP techniques, Universal Sentence Encoders, FastText, and BERT. Image data was transformed into dense representations using ResNet and convolutional autoencoders were included in the modeling. Recommendations were produced on an individual level using both matrix factorization and gradient boosting models.
How we did it
Preprocessing, training, validation, and inference pipelines were developed in Python on Azure. Primary tools/environments for the project were Snowflake, Databricks and Spark with orchestration through Apache Airflow. Model training was performed using various open-source libraries. Product text was utilized to provide even better recommendations, with feature extractors developed in TensorFlow.
—
Million SEK revenue
—
Employees
—
Online Growth Increase
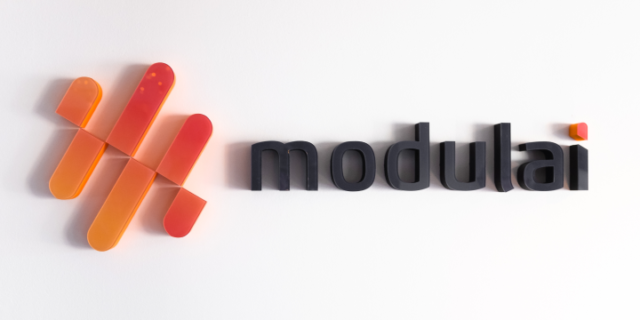
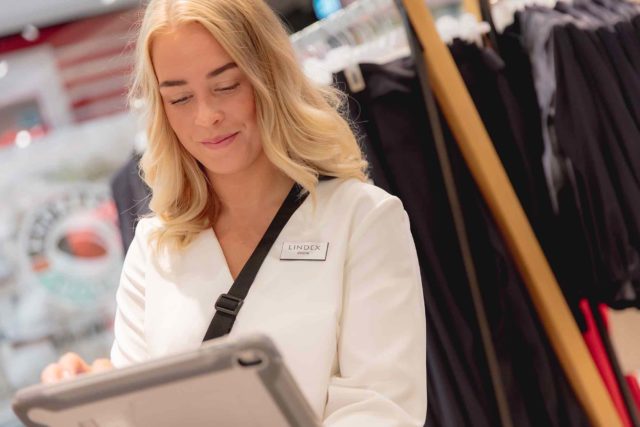
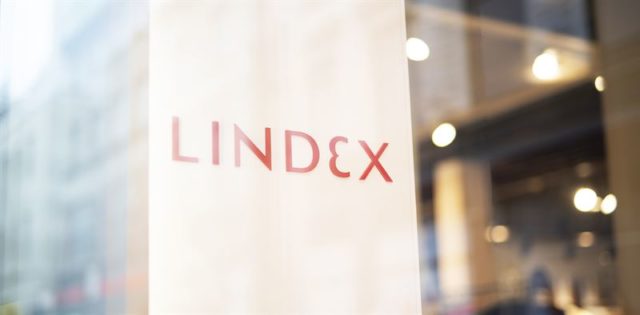
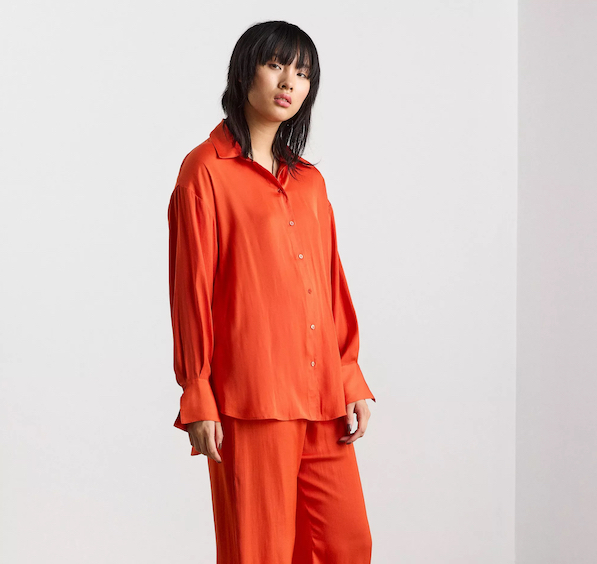